

A tale of two systems: how an AI-enabled public service impacts Human Learning Systems
Article highlights
This year, we joined partners to publish the #HumanLearningSystems e-book. Around the same time, @LorennRuster worked with us to explore the role of dignity in govt AI Ethics instruments. So we wonder, what is the role of AI in HLS?
Share article"Artificial Intelligence are technological systems, anchored in learning models, and created by, applied by and sometimes even emulating humans. AIs are not called Systems Learning Humans, but they could be." @LorennRuster
Share articleWhat are the implications of an AI-enabled public service on #HumanLearningSystems? And, how can HLS be applied to AI development processes? @LorennRuster explores in this @CPI_foundation article
Share articlePartnering for Learning
We put our vision for government into practice through learning partner projects that align with our values and help reimagine government so that it works for everyone.
Earlier this year, CPI joined a range of partners to publish an e-book describing a paradigm shift in the way that public management is conceived and carried out. Its ultimate goal is for governments to enable human flourishing. At its core, the emerging paradigm called Human Learning Systems (HLS), embraces a mindset that has humans at the centre, has a management strategy anchored in learning, and takes a systems view.
Around the same time, I was working with CPI on research centred on a similar goal of government-enabled human flourishing: in our case, how government frameworks guiding the development of Artificial Intelligence (AI) protect and promote human dignity.
It struck me that HLS and AI may have a few things in common. The Encyclopedia Britannica defines AI as:
The project of developing systems endowed with the intellectual processes characteristic of humans, such as the ability to reason, discover meaning, generalise, or learn from past experience.
In other words, Artificial Intelligences are technological systems, anchored in learning models, and created by, applied by and sometimes even emulating humans. AIs are not called Systems Learning Humans (as far as I know!), but they could be.
So I began to wonder about the role of AI in this emerging paradigm for government.
(Some of the) implications of an AI-enabled public service on HLS
For HLS to become the new norm in public management, it will need to co-exist with the world we find ourselves in. AI-enabled technologies, and the AI-enabled public service that emerges, are one part of our unfolding reality.
This topic will be considered primarily from the perspective of frontline public service workers who are, or will be, using AI-enabled tools as a part of their everyday roles. This could include social workers, health workers and/or people interacting with citizens trying to access government services. How might AI-enabled tools impact how frontline public servants implement an HLS approach?
AI-enabled tech as a part of a human-centred system of relationships
Humans and relationships are at the centre of HLS. The HLS e-book likens a human-centred system of relationships to a solar system. Seeing people in the context of a solar system is a way of understanding the aspects of a person’s life that contribute to them experiencing a positive or negative outcome. As a public servant, this is an important part of re-humanising what public management means, how it sees citizens and how it acts. The introduction of AI-enabled tools influences this solar system.
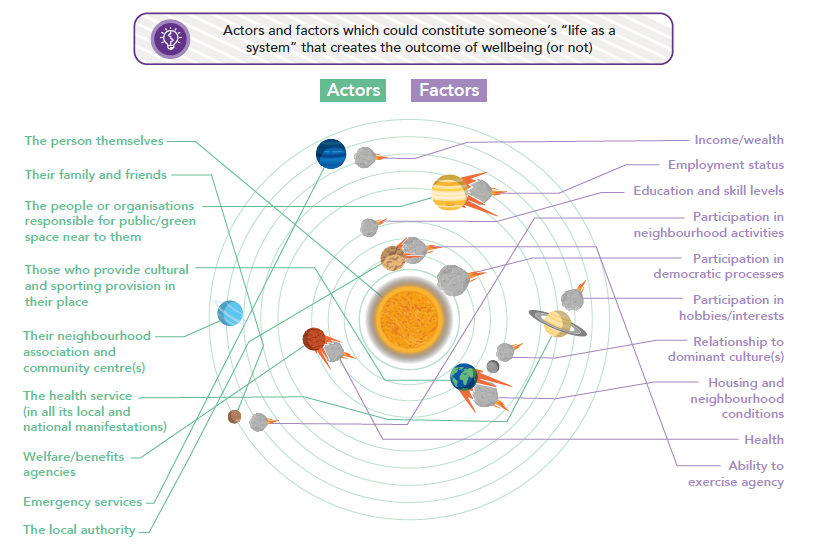
The person is in the centre and surrounded by different planets and different asteroids which interact over time. Planets represent the relationships an individual has with other people and other organisations (referred to as actors in HLS). Asteroids represent a bunch of other structural elements unique to the person that need to be taken into consideration such as their education, income/wealth, health, housing, ability to act and relationship with the dominant culture (referred to as factors in HLS). According to HLS, the planets and asteroids are interacting all of the time and these interactions create an outcome of wellbeing (or not) for individuals in the centre.
An AI-enabled public service can change people’s relationships with other people and organisations (actors). For example, let’s consider an AI-enabled chatbot which attempts to simulate human conversation either through text or voice (or both), picking up on cues such as sentiment. The chatbot forms the new interface of interaction with citizens and can be seen as an extension of people and organisations (like public servants and government departments) that would usually fulfil this function. At the same time, AI-enabled chatbots can act independently of humans in certain conditions, becoming actors in their own right.
As a public servant, understanding citizens’ interactions with other organisations or government departments through chatbots is a part of understanding their overall wellbeing. An AI-enabled chatbot could be considered as another planet (or actor) in the solar system of relationships.
AI could also have a role to play in the asteroids part of the solar system (i.e. as a factor). In a similar way to how a relationship to the dominant culture(s) can contribute to wellbeing, in an AI-enabled public service, consideration must be given to a person’s relationship to the dominant AI model(s).
For example, let’s consider a future AI-enabled chatbot that uses GPT-3: an artificial intelligence system currently in trial mode that generates text, mimicking human language. In addition to its creativity and human-like text, GPT-3 is also a system that has encoded bias and discrimination in the type of language it uses in response to particular cues. For example, researchers found that GPT-3 disproportionately uses language describing violence in response to text prompts that include the word ‘muslim’.
So fast-forward to a time where GPT-3 (or a version thereof) is included in a chatbot with this bias still intact. A person who is a Muslim would likely be impacted by the AI models in a different way to other populations. Although researchers are working on improving GPT-3 to minimise bias, it is nevertheless likely that frontline public servants will need to understand the potential relationship between individuals and the dominant AI models used as a part of their solar system of factors.
AI assisting and/or impeding shared sensemaking
In HLS, learning is the management strategy and crucial to all activities of public servants. The HLS report explains how public service management’s relationship with measurement has been focused on accountability and blame in the past, and needs to shift to aligning measurement with the goal of learning. So how might AI assist and/or impede this goal?
Shared sensemaking is identified in the HLS approach as a way to build trust through learning. This shared sensemaking is predicated on the existence of data -- and AI can assist with generating and analysing data. For example, let’s consider the case of the data produced in a smart city, managed by a local council. Smart cities generally involve sensors, collecting data on parking habits or levels of pollution in a particular city or a range of other factors.
This data can be used to train AI models and help public servants predict what actions to take in different circumstances. For example, pollution levels in a particular city can be connected to an understanding of traffic flows and assist in re-planning major thoroughfares through particular cities.
.png)
When put into collective hands, AI-enabled tools can assist in making sense of data and finding new patterns and correlations that would not be evident to a human observer. When packaged in ways people can understand, the data captured can be conducive to learning together, and building trust. At the same time, AI-enabled tools could also turbo-charge accountability and blame culture when not directed towards learning.
In the example of the smart city, pollution levels in a particular city could also be connected via the AI-enabled tool to factory emissions for example and lead to automated fines and community pressure to close down a business. Using AI-enabled tools in unrestricted ways instead of with a focus of learning, could further entrench feelings of surveillance, which ultimately erodes trust.
All in all, AI is neither good nor bad on its own when it comes to enabling a HLS agenda. Its intent and potential impacts are decided by humans. As such, public servants must consider the ways in which AI can be leveraged to build trust through shared sensemaking and be aware of how it may undermine trust-building efforts.
HLS applied to AI development processes
On the surface, HLS shares many similarities with AI - they both involve humans, are centred on learning and are systems. In practice, however, they couldn’t seem further apart. Given these connections, it seems plausible that the way of thinking in HLS might have some value for AI development processes. One such area of potential interest to AI development is HLS’s approach of thinking in scales.
Thinking in scales
According to HLS, a system is defined as a set of relationships between actors (people and/or organisations) and causal factors which combine to create a desired purpose (or ‘outcome’) in people’s lives. A system is an artificial boundary. And drawing that boundary is an act of power, as it demarcates the parts of the world that we choose to pay attention to.
In addition to thinking about the work they do as a system, HLS encourages public servants to think about the work they do at different levels of the system (also referred to as ‘scales’). Scales, in this context, can be thought of as levels or groupings that distinguish particular parts of a system. You can think of a system at the scale of a person/ individual, at the scale of a team or organisation, at the scale of a place, at the scale of a country, and many others.
Thinking in different scales involves:
identifying useful scales to begin with for example (person, team/ organisation, place, country);
identifying the actors involved at each scale (for example at the person level, actors could include members of the public / family / community and frontline public servants);
thinking about the impact of particular interventions at each scale for the actors involved; and
understanding the relationships between the different system scales.
Thinking in scales is a practice that, according to HLS, is needed not just once, but continually. But ultimately, why do it?
This approach of thinking in scales enables public servants to re-humanise their work by keeping proximate to the people involved. Proximity and human-centredness are also challenges faced by AI development and thereby may benefit from thinking in scales.
Thinking in scales also helps surface the different relationships that are important to the outcome that you’re wanting to achieve. In the case of designing an AI-enabled tool, it highlights that the AI tool is not operating in isolation, but in an emergent context of varied relationships that may undermine or assist with the intended outcome. Considering that tool at different scales is important for understanding positive and negative, intended and unintended consequences over time.
In HLS, thinking in scales also opens up a range of subsequent questions. When applied to AI development these questions could look like the following:
What is the purpose of the AI tool at each scale of the system? Do these purposes align across the different scales?
What is needed outside of the AI system itself for it to achieve its outcome? What does that look like at each scale of the system?
How are we stewarding the learning that occurs at each scale of the system? This includes both the management and governance of the AI tool.
Conclusions
The connections between HLS and AI are multi-faceted. As a frontline public servant, adopting an HLS approach occurs in a wider context that is constantly changing. AI-enabled tools are one part of this emerging environment, and as such, need to be overtly considered within the HLS approach.
Two ways in which this can be done are through active incorporation of AI as a part of the system of relationships, and through consideration of how AI may assist or impede shared sense-making. As a public servant designing, implementing, monitoring, or de-commissioning AI-enabled technology, an HLS approach is also of interest. Thinking in scales offers a way forward for both the public service and the AI it develops and uses, to re-humanise its approach, keep proximate to those impacted, and consider the wide range of relationships that will contribute to achieving desired outcomes.
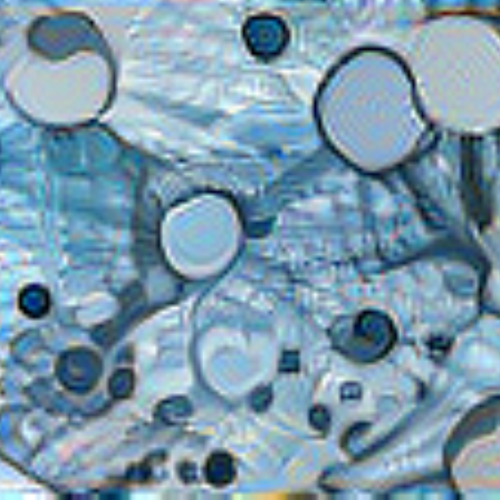
AI Ethics in Government
We believe that a core role of government is to create conditions for dignity for all. To do this well, governments need to ensure they are playing a role in both protecting and proactively promoting people’s dignity - we call this cultivating a Dignity Ecosystem.
Written by:
You may also be interested in...


Applying a dignity lens to government AI Ethics instruments: What does a dignity lens even mean?
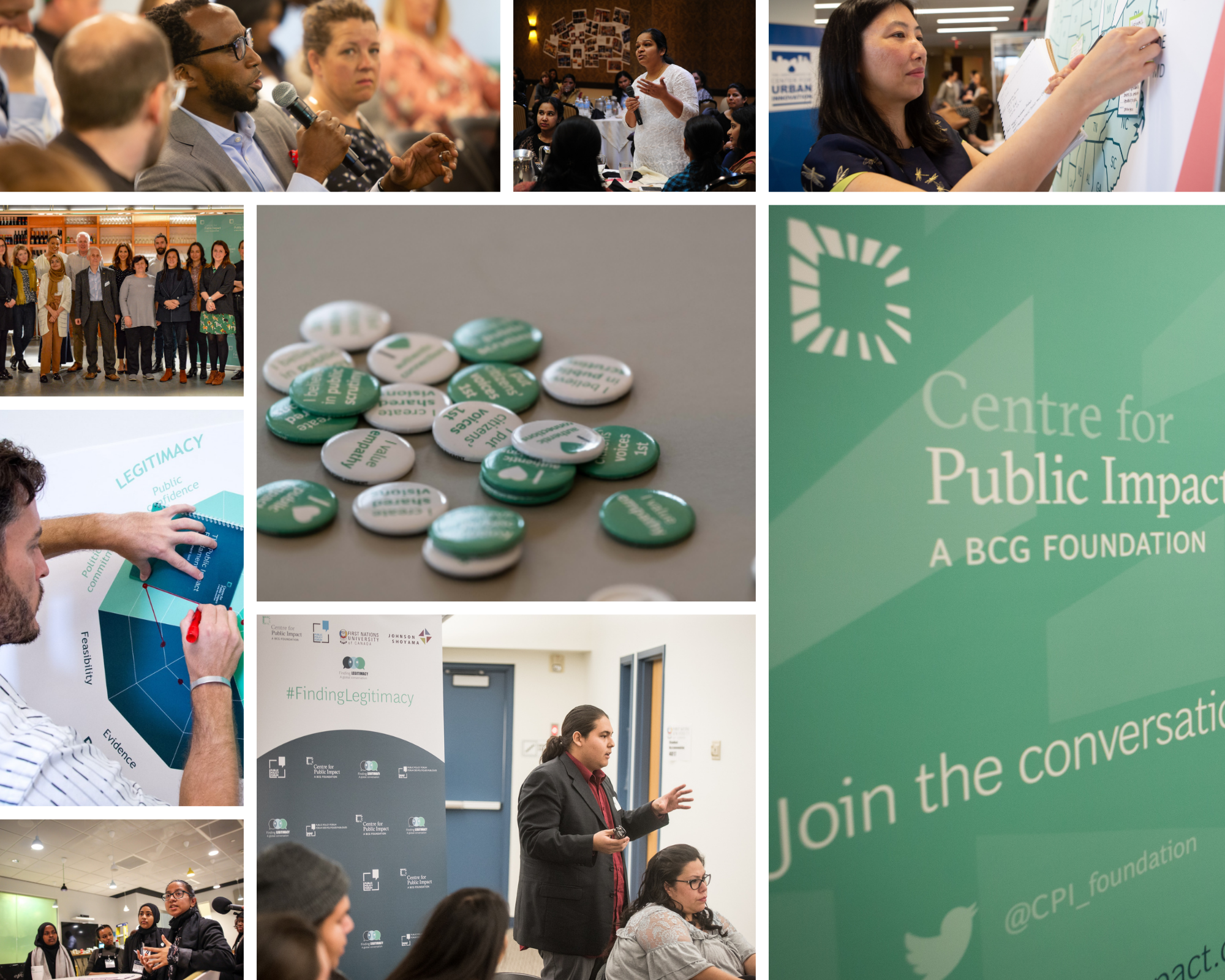